Old fictional representations of computers and robots presented them as entities of pure logic and rationality, incapable of any bias, prejudice and preference. Our understanding of machine intelligence has come a very long way since then, and we know today that prejudice is a lot more difficult to keep out of binary processes than we initially imagined.
A wealth of academic and popular literature, most famously epitomised in Cathy O’Neil’s 2016 book Weapons of Math Destruction, has demonstrated that human prejudice tends to get encoded into the machines we build – including gender bias.
This doesn’t affect only the conditions and the representations of women in the tech industry specifically, but extends to the perpetuation of the entire spectrum of stereotypes deployed against women in culture at large. Artificial Intelligence (AI) first learns these stereotypes by encountering them in its databases, and then reiterates them in its production.
In this article, we will explore the unique problem of gender bias in AI, and the difficult question of how to overcome it – a question which, as we will see, involves not just the tech industry but all of us both individually and socially.

How does AI develop gender bias?
There are two primary sources of sexist prejudice in AI.
The first is endocentric, meaning that it results from the engineering process of the AI itself. Tech teams that lack gender diversity tend to write code and parameters that ignore the perspectives and preferences of minorities, and the final result is an AI that ‘thinks’ like a group of men (typically white, straight, able-bodied men).
Worse, programmers with conscious sexist prejudice may encode their own prejudice into the systems they create, so that when training the system to understand the concept of ‘doctor’ they only provide pictures of men, while when training the system to understand ‘nurse’ they may only provide pictures of women.
The second source of bias is exocentric, meaning that it has to do with the external models that an AI system will learn from. Even if a programming team is diverse and does not inject its own prejudice into its system, if the system is trying to learn about ‘action movies’ and almost all of the movies it encounters have male protagonists, it will ‘learn’ that women cannot be action heroes.
This second problem is unfortunately much harder to fix, as it would mean removing sexist prejudice from the entire world – a worthwhile endeavour no doubt, but more than a little beyond the scope of this article!
Nonetheless, the first and the second problem are linked. As we will see, a more diverse team can do much to address the bias in their data.
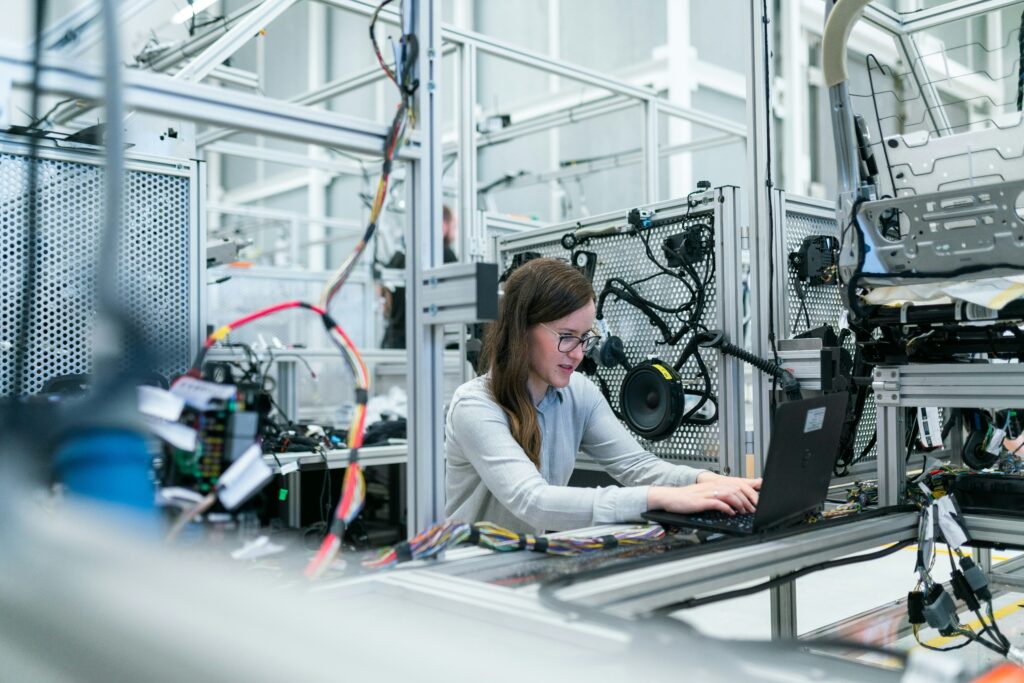
Overcoming gender bias: the engineer’s problem
It should come as no surprise that the first responsibility for AI creators is to employ teams that are as diverse as possible, and – crucially – having diversity in leadership roles, so that different human intelligences may contribute to the shaping of the artificial one. This doesn’t mean only gender diversity but also diversity in terms of ethnicity, culture, sexual orientation, and ability.
Even with a diverse team, efforts must be made at the engineering level to ensure the AI system is fed a representative, diverse dataset, and where this is not possible, that it does not accidentally learn bias from it. This means encoding into the AI the ability to learn about human roles, tasks and activities without associating them to any gender.
The above responsibilities are fairly intuitive. One that is not as readily understood is the problem of algorithmic transparency: the way that an AI makes decisions should be one that can be monitored, seen, and explained. This is a much more complex engineering challenge than it may initially appear, as it is impeded by the notorious ‘black box’ problem in AI, but it is key to patching artificial gender bias where it manifests.
Finally, overcoming gender bias in AI will require not just tech professionals but regulators to step up. The good news is that there seems to be an appetite for greater AI regulation, with the European Union, the United States and the United Kingdom all having taken steps in that direction. The bad news is that most of this effort is presently too general in form to address gender discrimination directly, and will require very considerable progress and refinement.

Overcoming gender bias: the user’s problem
There is an unfortunate tendency to dismiss gender bias in AI as a problem for tech companies and governments only. In reality, as AI becomes more and more integrated into our everyday lives, using it responsibly should be seen as everyone’s problem.
As a user, do not just assume that your AI will be unbiased. Instead, ward against discrimination actively: if you use AI for personal evaluation (for example, filtering CVs to assess candidates for a position) then encourage blind testing, removing name and picture from the parameters that the AI can read.
As well, phrase prompts with inclusion in mind. Don’t just ask Midjourney to give you a picture of ‘6 engineers evaluating a project’, rather ask for ‘6 engineers, 3 men and 3 women, evaluating a project’.
The same principle should apply when prompting for text or any other kind of content, and this is especially important for ‘template prompts’ – that is to say, prompts you use over and over with only slight modifications to produce iterable content.

A shared responsibility
Only 10 years ago, AI was a domain exclusive to a handful of forward-looking specialists in the world of tech. Today, as it becomes an integral part not just of our industries but of our everyday lives, AI is increasingly becoming a responsibility of us all.
Gender bias in AI is a problem that has been recognised and discussed in academia for several years, but so far solutions have been demanded only from the tech industry and the legislators. Yet one of our core beliefs at WBS CODING SCHOOL is that tech does not move forward in isolation – it only goes where we choose to take it.
AI will only become fairer, to women and to men alike, when all of us start thinking fairer. Overcoming gender bias in AI is a shared responsibility that does not end at the gates of the tech world, and which certainly does not end with this article.